Micron Kicks Off Production of HBM3E Memory
by Anton Shilov on February 26, 2024 1:00 PM EST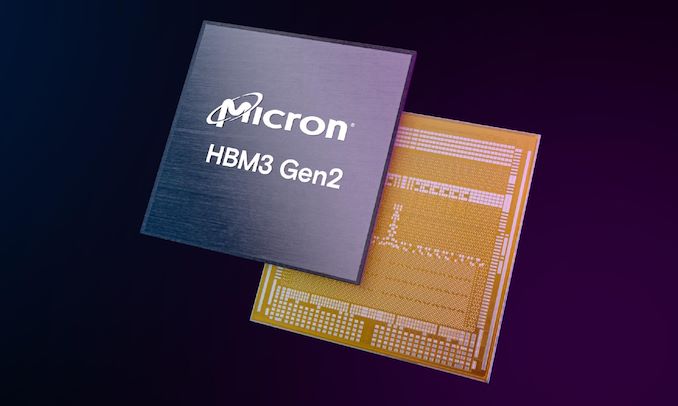
Micron Technology on Monday said that it had initiated volume production of its HBM3E memory. The company's HBM3E known good stack dies (KGSDs) will be used for Nvidia's H200 compute GPU for artificial intelligence (AI) and high-performance computing (HPC) applications, which will ship in the second quarter of 2024.
Micron has announced it is mass-producing 24 GB 8-Hi HBM3E devices with a data transfer rate of 9.2 GT/s and a peak memory bandwidth of over 1.2 TB/s per device. Compared to HBM3, HBM3E increases data transfer rate and peak memory bandwidth by a whopping 44%, which is particularly important for bandwidth-hungry processors like Nvidia's H200.
Nvidia's H200 product relies on the Hopper architecture and offers the same computing performance as the H100. Meanwhile, it is equipped with 141 GB of HBM3E memory featuring bandwidth of up to 4.8 TB/s, a significant upgrade from 80 GB of HBM3 and up to 3.35 TB/s bandwidth in the case of the H100.
Micron's memory roadmap for AI is further solidified with the upcoming release of a 36 GB 12-Hi HBM3E product in March 2024. Meanwhile, it remains to be seen where those devices will be used.
Micron uses its 1β (1-beta) process technology to produce its HBM3E, which is a significant achievement for the company as it uses its latest production node for its data center-grade products, which is a testament to the manufacturing technology.
Starting mass production of HBM3E memory ahead of competitors SK Hynix and Samsung is a significant achievement for Micron, which currently holds a 10% market share in the HBM sector. This move is crucial for the company, as it allows Micron to introduce a premium product earlier than its rivals, potentially increasing its revenue and profit margins while gaining a larger market share.
"Micron is delivering a trifecta with this HBM3E milestone: time-to-market leadership, best-in-class industry performance, and a differentiated power efficiency profile," said Sumit Sadana, executive vice president and chief business officer at Micron Technology. "AI workloads are heavily reliant on memory bandwidth and capacity, and Micron is very well-positioned to support the significant AI growth ahead through our industry-leading HBM3E and HBM4 roadmap, as well as our full portfolio of DRAM and NAND solutions for AI applications."
Source: Micron
8 Comments
View All Comments
QChronoD - Monday, February 26, 2024 - link
One of these stacks has more bandwidth than a 4090. It probably wont be until the 6000 series that we start seeing older gen HBM being used on consumer cards.PeachNCream - Monday, February 26, 2024 - link
Unlikely. Production will shift to newer generations of HBM and leave no plant space or production capacity for prior generations. HBM will have to be cost effective for consumers in whatever generation is current before it makes it way into the mainstream.HideOut - Monday, February 26, 2024 - link
Correct, in fact AMD DID release a disasturous card a few years ago using HBM. Its the only consumer card with it. We'll probably never see it again.Threska - Monday, February 26, 2024 - link
Still rockin my Vega.Orfosaurio - Monday, February 26, 2024 - link
More like "cost-effective enough"...zepi - Tuesday, February 27, 2024 - link
So far Nvidia's AI hype has been eating all interposer packaging capacity at TSMC, so there has been no spare capacity to sell any GPU + HBM / interposer combinations for consumers - irrespective of HBM generation.Maybe one day this will change - but Nvidia will still need to make sure that they don't sell too big memories / memorybandwidths for gamers so that they would end up being used as replacements for the most expensive datacenter GPU's.
Papaspud - Tuesday, February 27, 2024 - link
I live near where Micron is headquartered, they are building a massive new chip plant= $15 billion, should be online next year...not sure if it will be making this memory though. Maybe memory prices will drop.Sudionew - Monday, April 8, 2024 - link
Curious to see the performance improvements in real-world applications. Hopefully, this translates to faster training times and better accuracy for AI models.